Understanding the Dynamics of Large Language Models in Behavior Change
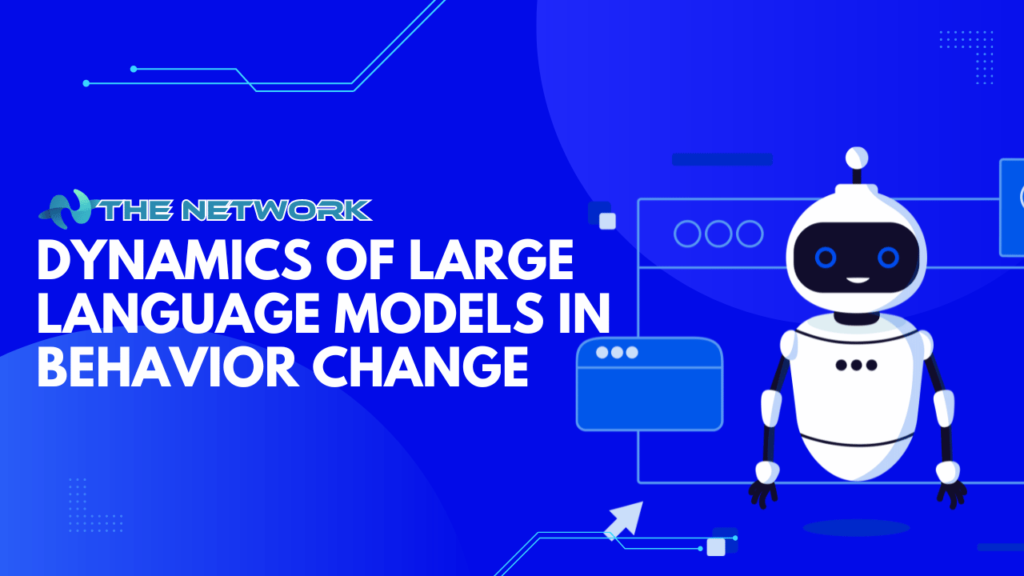
In the realm of artificial intelligence (AI), large language models (LLMs) have emerged as transformative tools, particularly in healthcare and education. However, a recent study by researchers from the University of Illinois Urbana-Champaign sheds light on a critical aspect: while LLM-based chatbots can be effective in supporting behavior change for individuals ready to act, they struggle to recognize and address users’ motivations when they are hesitant or ambivalent. This blog post explores the nuances of this research, delving into the types of language models for AI chatbots, the reasons behind LLMs’ inability to effectively recognize motivations, their advantages and disadvantages, future applications, and practical implications for education and parenting.
Types of Language Models for AI Chatbots
Language models for AI chatbots vary in complexity and functionality. Traditional rule-based models follow predetermined patterns, while statistical models rely on probabilities to generate responses. More advanced models, such as transformer-based LLMs like ChatGPT, Google Bard, and Llama 2, utilize deep learning techniques to understand and generate human-like text. These models differ in their architectures, training data, and capabilities, with transformer-based models demonstrating superior performance in natural language understanding and generation.
Â
Why LLMs Struggle to Recognize Motivation
LLMs face challenges in recognizing users’ motivations due to their training data limitations and inherent design. These models excel in representing the relevance of user language but lack comprehension of nuanced motivational states. Users’ queries often lack semantic differences across motivational stages, making it difficult for LLMs to discern between hesitation and commitment to action. Additionally, LLMs’ inability to engage emotionally and provide tailored support further hinders their effectiveness in addressing early-stage motivational barriers.
Â
Top Talking Points
- Limited Recognition of Motivational States: LLMs fail to identify users’ motivational states accurately, particularly in the initial stages of behavior change when individuals are hesitant or ambivalent.
- Effective Support for Ready-to-Act Users: Despite their limitations, LLMs can effectively support users who have established goals and are committed to behavior change, providing relevant information and guidance.
- Emotional Engagement and Readiness: LLMs struggle to generate emotional engagement and readiness for change, crucial factors in overcoming motivational barriers and sustaining behavior change.
- Tailored Support and Information: Users in different motivational stages require tailored support and information. LLMs often fall short in providing contextually relevant guidance, impacting their ability to facilitate meaningful behavior change.
- Future Directions in Model Development: Future research aims to refine LLMs by incorporating linguistic cues, information search patterns, and social determinants of health to better understand users’ motivational states and provide personalized support.
Â
Advantages and Disadvantages of LLMs
Advantages:
- Enhanced accessibility to information and support.
- Scalability in reaching a large user base.
- Potential for personalized interactions and guidance.
Disadvantages:
- Limited understanding of nuanced user needs and motivations.
- Inability to provide emotional support and tailored guidance.
- Ethical concerns regarding data privacy and algorithmic biases.
Â
Future Applications of LLMs
The potential applications of LLMs extend beyond healthcare to education, customer service, and entertainment. These models can revolutionize personalized learning experiences, streamline communication channels, and enhance user engagement in various domains.
Â
Key Facts
- LLM-based chatbots show promise in promoting behavior change but struggle to recognize users’ motivations effectively.
- Users’ motivational states vary across different stages of behavior change, posing challenges for LLMs in providing tailored support.
- Traditional language models, such as rule-based systems, lack the sophistication to understand and generate human-like text compared to transformer-based LLMs.
- Future research aims to refine LLMs by integrating linguistic cues and social determinants of health to better support behavior change initiatives.
- The ethical implications of LLMs in influencing user behavior and decision-making raise concerns regarding data privacy and algorithmic biases.
Â
School or Homeschool Learning Ideas
Â
- Role-play Activity: Students can role-play scenarios representing different motivational stages of behavior change, emphasizing the importance of empathy and understanding in providing support.
- Case Studies: Analyzing real-life case studies of individuals undergoing behavior change journeys can deepen students’ understanding of motivational barriers and effective intervention strategies.
- Interactive Workshops: Hosting interactive workshops on goal-setting and behavior change can empower students to identify their motivations and take proactive steps towards achieving their objectives.
- Digital Storytelling: Encouraging students to create digital stories depicting personal experiences with behavior change can foster creativity and self-reflection.
- Peer Support Groups: Facilitating peer support groups where students share their challenges and successes in behavior change can promote collaboration and mutual encouragement.
Â
What Our Children Need to Know
- Understanding Motivation: Children should learn about the different stages of behavior change and how motivation influences decision-making and actions.
- Empathy and Support: Teaching children to empathize with others’ struggles and provide support without judgment cultivates a supportive and inclusive environment.
- Critical Thinking: Encouraging children to critically evaluate information and seek diverse perspectives fosters informed decision-making and resilience in navigating behavioral challenges.
Â
The Big Questions
- How can we balance the benefits of AI in behavior change with the ethical concerns surrounding data privacy and algorithmic biases?
- What role should schools play in educating students about the potential risks and benefits of interacting with AI-powered chatbots for behavior change?
- How can we ensure equitable access to AI-driven support systems for behavior change across diverse socio-economic backgrounds?
- What strategies can parents implement to complement AI-based support tools in guiding children through behavior change processes?
- How might advancements in AI technology reshape the future landscape of healthcare, education, and personal development?
Â
Conclusion
In conclusion, while large language models hold immense potential in supporting behavior change initiatives, their current limitations in recognizing users’ motivations underscore the need for continued research and development. By addressing these challenges and fostering interdisciplinary collaboration, we can harness the power of AI to empower individuals in their journey towards positive behavior change.
Responses